#008 - One step closer to Skynet
You're reading Complex Machinery, a newsletter about risk, AI, and related topics. (You can also subscribe to get this newsletter in your inbox.)
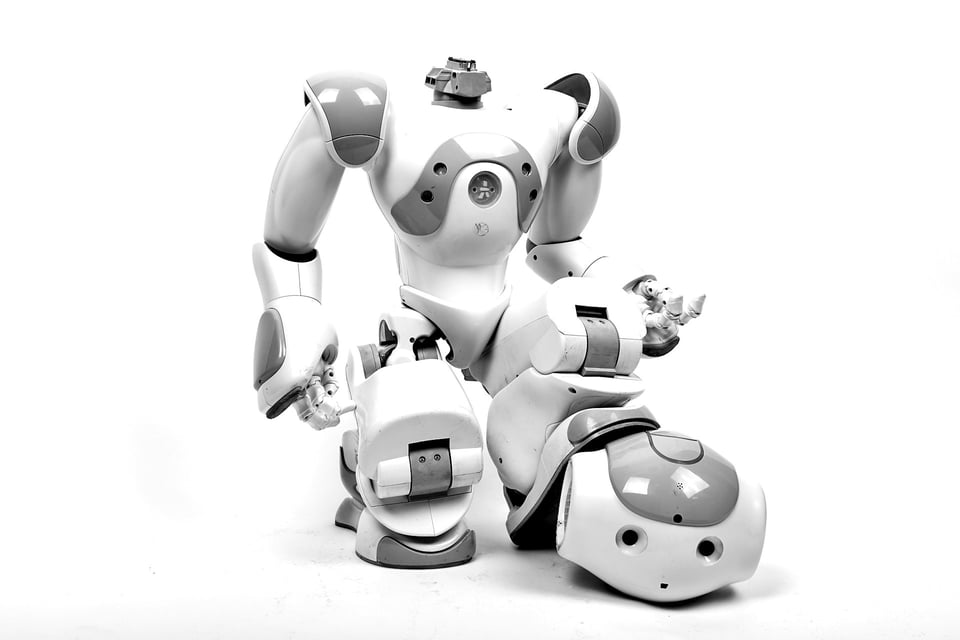
Last month I noted four ways that the AI field is sabotaging its image. This time around I'll share two recent examples of Fake It Till You Make It, and a tangential connection to When It Works Too Well.
GPT-4Loko
I believe it was Guillermo Roditi Domínguez (New River Investments) who summarized algorithmic trading as doing battle with the world's largest random number generator.
There's a lot of truth there. As a trader you realize that your job is not just to survive in that twisted, complex adaptive system of human decision-making and machinery, but to somehow come out ahead. The whole time a variety of Weird Stuff™ – in the market, in your brain, in your code – is ready to wipe you out. So you test the hell out of everything. You build in padding and safeguards. And then you carefully watch the machines as they operate in the wild.
I've been thinking about that in light of the latest OpenAI model release, dubbed GPT-4o. (Yes, that's a number 4 and a lowercase letter o. Their spelling, not mine.) It's supposedly faster, smarter, and cheaper than its predecessors. We've come one step closer to Skynet.
We've also taken one step back.
GPT-4o is still pretty dumb in some ways. Eerily dumb. Yet so self-assured. Consider these interactions (shared with permission) in which fellow AI professional Russell Horton put GPT 4o to the test:
prompt:
Think of a a major American corporation of the past (two words, 15 letters altogether).
Change the last three letters in the second word
the resulting phrase will name something that will occur later this year. What is it?
GPT-4o response:
The major American corporation is "General Motors."
Changing the last three letters of "Motors" to "2024" results in "General 2024," which implies a "general election," an event that will occur later this year.
Hmm. And then:
prompt:
Think of a popular online service. Change the first letter to a Y and rearrange the result to get what this service provides.
GPT-4o response:
The popular online service is "Gmail."
Changing the first letter to a Y gives us "Ymail." Rearranging the letters of "Ymail" results in "Mail," which is what the service provides.
Elsewhere I've seen screencaps of 4o stumbling over slight twists on well-known riddles.
Why does this new, fancy release of ChatGPT do this? Mostly because it has no idea it is wrong. It doesn't have a concept of right or wrong, or even fact, as it only parrots linguistic patterns it picked up looking at (numeric representations of) words in its training data. Hence why the model's responses are grammatically correct even when they are complete nonsense.
Does this mean 4o is bad? No.
Well, I mean … sort of.
These goofs are reminders that GPT-4o is an AI model. A big pile of linear algebra in fancy packaging. And like every AI model, 4o will be wrong now and then. Sometimes in subtle ways that we don't notice. Right or wrong, a model will deliver answers with the same bold, misplaced confidence of a straight man arguing on the internet.
Companies in the pre-GPT era learned this the hard way because they had to invest tons of money into data science teams and expensive computers in order to use AI. Nowadays anyone who can type into a text box can see first-hand how, and how often, the bots stumble. The popularity of genAI chatbots is, weirdly, teaching people some truths applicable to all of AI. Not my preferred path to widespread AI literacy but I'll take it.
Unlike their predictive cousins, though, generative AI models have an extra dose of The Random™ . That extra something that takes them down weird pathways. Working with genAI reminds me of my days doing concurrent software development: hard to get right, but easy to look like it's working when it is not. Every day is a sea of close calls that you never knew about.
If the financial markets are the world's largest random number generator, then genAI models run a close second or third. And since companies insist on using genAI for customer service, evaluating job candidates, writing code, or any number of other tasks to which it has already proven barely suitable, then maybe they can look to algorithmic trading for ideas on safety? That would at least limit the scope of damage.
I was about to end that segment with "food for thought" but then this came across my desk:
No thanks, I'm on a glue-free diet
Speaking of bots that lied their way through the job interview, Google's AI Overview search summary tool has suggested using glue as an adhesive for pizza cheese. It also says that people should eat more rocks. And that a splash of gasoline will really spice up tonight's spaghetti. Penne alla benzina, anyone?
As bad as these are – and they're very bad, to be clear – Google is late to the game. GenAI bots offering poison recipes is so last year.
AI Overview's core problem is that it treats facts, internet snark, and conspiracy theories with equal reverence as it builds its summaries. (Case in point: the pizza-glue idea was traced back to an old Reddit post.) Does this sound familiar? It's the web search cousin of YouTube's recommendation rabbit-hole. The same reason why you watch a YouTube video from The Financial Times, and then it offers you more FT videos shoulder-to-shoulder with title-in-all-caps crypto-bro doom rants.
All of this leads me to my wider concern, that Google should be better than this. The company has tons of in-house AI talent, plus years of experience to match. Google should be at the forefront of corporate AI use cases, giving us reason after reason to have faith in what AI can achieve. Instead, it's been rushing to jam AI into everything it does and rolling out weak excuses when the things inevitably fail. CEO Sundar Pichai, for example, simply claims that the hallucinations in AI summaries are "still an unsolved problem."
If Google needs AI Overview to discern fact from fiction, and it is unable to discern fact from fiction, then it sounds like the "solution" would be to pull the plug?
Small thinking in a big world
A product's development lifecycle should include some "What if ..?" questions. Call it a risk assessment or an impact assessment or a simple brainstorming session. The idea is that you uncover potential problems early and adjust accordingly. Like, say, if you were an artist who wanted to build a public video livestream that connected New York City to Dublin, you could ask "What if people are their usual nasty selves? How could I build in some safeguards?"
I've worked in tech long enough to know how often companies skip over that step. Which is why I wasn't surprised to read that AI startup ElevenLabs was so busy building a tool to clone human voices that forgot to add basic safety mechanisms on the first go-round. And when they finally go around to that,
[...] the safeguards from ElevenLabs were "half-assed," Hany Farid, a deepfake expert at UC Berkeley, told me--an attempt to retroactively focus on safety only after the harm was done. And they left glaring holes. Over the past year, the deepfakes have not been rampant, but they also haven't stopped.
I can imagine how greed shareholder value might convince a company to skip the risk assessment. But it's not just greed at play there. There's also the tunnel vision that comes with only thinking about the technology. And if you can get past this article's clickbait subtitle – "the case for teaching coders to speak French" – you'll see what I mean.
The subtitle did its job in drawing me in. First because of the overall concept – hey, I speak French and I've written a lot of software in my time; maybe there's something here for me! – and second because of that word "coder" in the middle.
I bristle when I hear that term. I first came across it in early 2000s job postings, where it was usually specified as "heads-down coder." These companies wanted someone who would think about churning out code and nothing more. I found it just as offensive as its cousin, "code monkey," because it made it clear that the most desirable software developers knew very little about the world around them. And didn't care to know, either.
That takes us back to the article. Author Ian Bogost points out that modern computer science education is very much aligned with the Only Needs To Know Code approach. Which has led to some (un?)intended consequences:
But the computing industry isn’t just fast-moving. It’s also reckless. Technology tycoons say they need space for growth, and warn that too much oversight will stifle innovation. Yet we might all be better off, in certain ways, if their ambitions were held back even just a little. Instead of operating with a deep understanding or respect for law, policy, justice, health, or cohesion, tech firms tend to do whatever they want. Facebook sought growth at all costs, even if its take on connecting people tore society apart. If colleges of computing serve to isolate young, future tech professionals from any classrooms where they might imbibe another school’s culture and values—engineering’s studied prudence, for example, or the humanities’ focus on deliberation—this tendency might only worsen.
Someone who only thinks about code will be great at finding code problems. But less so at spotting bigger-picture issues, like how their work might cause trouble beyond its intended purpose.
Take AirTags. They're great for locating your lost items! And also for stalkers to track people. So-called "smart home" devices? They give a high-tech edge to domestic abuse. Facial recognition is good for … well, not much. But it's really popular despite that it doesn't really work.
How many people on those teams were thinking beyond the code? (Not many, it seems.) And will today's AI startups learn from past tech blunders? Because if their vision is so narrow that they can't ask a decent "What if…?" question – like, say, "What if people clone the voices of politicians to spread misinformation?" – then the rest of the world will be left to ask: "What the hell just happened? And why won't it stop?"
In other news …
- Last year, a magazine "interviewed" an AI clone of Formula One racer Michael Schumacher. The driver's family has successfully sued the publication. (ESPN)
- Reddit will grant OpenAI access to its data. I guess they inked this deal before The Pizza Glue Incident? (The Verge)
- Automation should make our jobs easier. But not always. (Vox)
- Turning to synthetic AI audience profiles in lieu of, y'know, surveying actual people. (Le Monde)
The wrap-up
This was an issue of Complex Machinery.
Reading online? You can subscribe to get this newsletter in your inbox every time it is published.
Who’s behind Complex Machinery? I'm Q McCallum. I think a lot about AI and risk, which I write about here.
Disclaimer: This newsletter does not constitute professional advice.