#024 - AI's last-mile problem
Robots start the job, but people finish it.
You're reading Complex Machinery, a newsletter about risk, AI, and related topics. (You can also subscribe to get this newsletter in your inbox.)
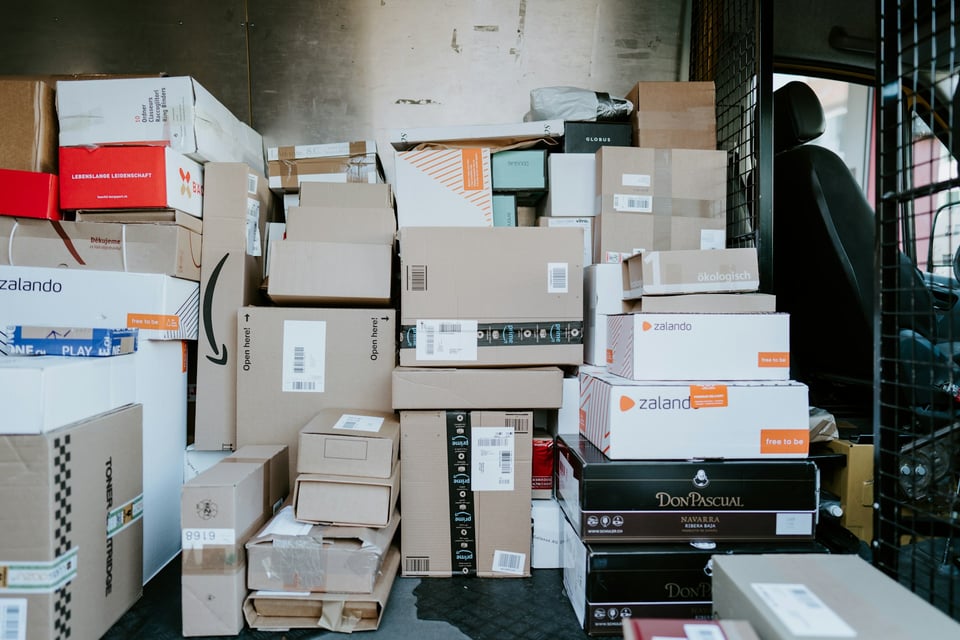
One fun part of writing is when you get an idea for a piece, then it starts to sound eerily familiar, and then you unearth a detailed outline you wrote three years ago.
(The lesson to all you writers out there: Finish. Your. Damned. Drafts.)
My most recent such experience started with an article on warehouse robots by Peter Eavis. I've thought a lot about workplace automation over the years – everything from where AI is a good or bad fit, to how we need to support the people whose employment is at stake – but Eavis's piece jogged my memory on one particular aspect of automation: the dreaded last-mile problem (LMP).
One mile, lots of steps
Even if you've never heard the term, you're familiar with the concept. Have you ever seen a process that works well at scale, but frays at the tail end? That last part, that need for messy precision just before the finish line, is the last-mile problem.
To mark yourself as a member of A Certain Generation, your introduction to LMPs came from the phone company or ISP. The provider could manage most of the network service from central locations, but extending that network into your home – closing the "last mile" from the central spot to the residence – required a technician's visit. (And an all-day wait window in some cases.) The same story plays out today with the warehouse model popularized by Amazon: a retailer can mass-ship packages between their facilities, but eventually that mass splinters off into individual, house-to-house deliveries.
I refer to LMPs as a "dreaded" problem because they erode the efficiencies you gained by doing work in bulk. You go from One Problem With One Solution to Millions Of Tiny Problems, All Of Which Need Individual Attention.
Simple solutions
For the most part, you address a last-mile problem by throwing people at it. (Figuratively speaking, of course.) That's why online retail employs armies of delivery drivers. And speaking of armies, LMPs exist even in war. The ideal would be to handle matters with a drone strike from altitude or artillery barrage fired from offshore, but certain operations require more precision. You have to send in the infantry to get up-close and personal with the objective.
Sometimes you can shift the inefficiency to the recipient. Notice how Amazon has created centralized lockers and Pickup locations. They still get to deliver to their facilities in bulk, and you as the customer make the (usually, short) hike to that same location. You become your own last-mile delivery service.
You can also change what you deliver. Netflix did this when it introduced streaming. Movies still needed to travel from Netflix's server farm to individual customer devices, but at that point it shifted from being a postal logistics problem to an internet connectivity problem. Which made it an ISP problem, not a Netflix problem.
The common thread here is that last-mile problems rarely disappear outright. You have to transfer the burden elsewhere, and in most cases that amounts to a fleet of people to handle the fiddly bits. Eventually enough of the fiddly bits have so much in common that they become a problem you can solve in bulk. But you don't have many other options until then.
Bots (including those made of bits)
That brings us back to warehouse robots. As Eavis points out, they're great for certain things. But some tasks require a human touch:
There are many crucial, simple tasks that humans are far better at. They can reach into a container of many items and move some out the way to extract the piece they want, a task industry officials refer to as picking. Robotics engineers struggle to say when their creations will be able to do that fast enough to be viable replacements for human workers.
Seen through the eyes of LMPs, warehouse robots represent the centralized, scalable part of "delivery" (locating items on shelves and moving them about the facility) and humans handle the last mile that involves precision (picking out smaller items, putting those items in boxes).
Right now there are too many of those fine-grained tasks for researchers to work on, and they're not economically feasible to solve. But over time the robots' speed and precision will improve and their costs will fall, making them amenable to a larger set of problems. That will shrink the last-mile space currently occupied by human labor.
What holds for warehouse robots holds for other technology-driven automation. Code and AI are great at handling clear, well-defined, very specific tasks that you need to perform in bulk: "complete this transaction," "classify this document," "evaluate this home price." But they don't do as well outside of those boundaries. It helps to think of tech automation as warehouse robots made of code and linear algebra. Just as that warehouse robot is not very adaptable, nor are software applications or AI models. And that means, for now, the leftover last-mile tasks will fall to people.
Plus ça change …
Oh, that old paper outline I found? The one I cobbled together in 2021? It went into detail on different last-mile problems, and ended with a section on AI. This was when the term "AI" meant "ML/AI," like predictive models. The core message – that AI breaks down on last-mile problems – was true three years ago and still holds for today's genAI.
Be mindful of anyone who claims that AI will handle a process end-to-end. (Doubly so, when this person is trying to sell you on their AI-backed product.) Those processes likely entail some form of a last-mile problem, in which case you'll have to sort out the last mile of the journey on your own.
In other news …
I'll start with two more articles on warehouse robots:
"Amazon’s New Robotic Warehouse Will Rely Heavily on Human Workers" (WSJ)
"Agility Robotics CEO tells BI how its humanoid robots are entering the workforce -- and getting paid for it" (Business Insider)
And then the rest of the news:
Is it time to change popular AI benchmarks? (MIT Technology Review)
Letting the robot speak on your behalf. Or role-play other people for you. Sort of. (The Guardian)
Is that someone you know? Or an AI imitator? Here's how to trip up the clone. (Ars Technica)
Ad agencies embrace AI. (Le Monde 🇫🇷)
AI companions continue to cause trouble. Including one from a Google-backed startup. (Futurism and Washington Post)
OpenAI's latest model has been a little dishonest. Proof that it's becoming more human? (TechCrunch)
If having access to AI is bad for kids, the only thing worse is … kids losing access to AI? (Aftermath)
Want a cool job in AI? Head for a bank. No, seriously. (WSJ)
The wrap-up
This was an issue of Complex Machinery.
Reading online? You can subscribe to get this newsletter in your inbox every time it is published.
Who’s behind Complex Machinery? I'm Q McCallum. I think a lot about AI and risk, which I write about here.
Disclaimer: This newsletter does not constitute professional advice.