#032 - What the machines don't know
Incomplete information can make AI models overly optimistic – even arrogant.
You're reading Complex Machinery, a newsletter about risk, AI, and related topics. (You can also subscribe to get this newsletter in your inbox.)
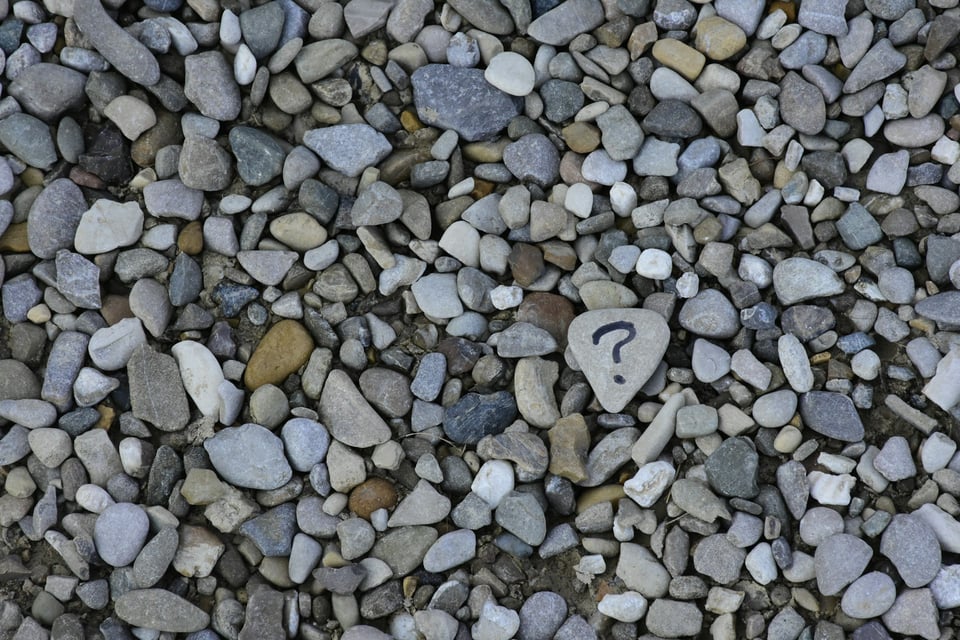
The arrogance of ignorance
Earlier this year, Apple's AI system took some well-deserved flak for mis-summarizing news headlines – making the summaries appear to have come from the news outlets in question, no less. For its next act, Apple Intelligence will group and sort your app notifications:
[The iOS 18.4 developer beta] adds a new “Priority Notifications” feature, powered by Apple Intelligence. The addition aims to help users manage their notifications by prioritizing important alerts and minimizing distractions from less important ones.
These priority notifications are displayed in a separate section on the phone’s Lock Screen. Apple Intelligence will analyze which notifications it believes should be shown in this section, but you can still swipe up to view all of your notifications.
The key word there is "believes." Not "knows." Remember GMail's priority inbox feature? And the way LinkedIn keeps prodding you to connect with randos? All of this reminds us that purveyors of AI tools don't know how we would decide. They can only guess. They claim it's an educated guess, because it is based on mathematical analysis of data. But that education stops short of a diploma.
To be fair, every ML/AI model will be wrong some percentage of the time. You'll never perfectly map real-world phenomena to a mathematical formula. But the models suffer from another weakness: their predictions are based on incomplete information.
LinkedIn can infer some things about me, for example, but I have professional relationships that exist beyond the eyes and ears of the platform. (LinkedIn has never suggested that I connect with these people, either. When you consider the number of complete strangers the platform has claimed I might know, that's telling.) Similarly, Gmail can take a guess as to which messages might be important to me. But since it doesn't have the full scope of my life, its models are a poor substitute for my decision-making apparatus.
These systems are uninformed strangers pretending to be your friend. They opine with confidence, despite operating on woefully incomplete information. They are arrogant in their ignorance.
With that, I hereby declare "arrogance" to be the collective noun for AI.
Say it out loud:
An arrogance of AI models.
Has a nice ring to it, no?
Return of The Random™️
A year ago, I warned about a little something I call The Random™️. It’s the wild animal inside every genAI bot that eventually breaks out and causes trouble. The take-away lesson? Companies need to either keep an eye on those LLMs, or stick to using them in low-stakes environments.
The LA Times chose a third path – letting their bot run free, providing color commentary alongside the site's articles. If you just winced when you read that line, it's because you could tell what came next: The Random™️ made an appearance. It took the form of your overly-opinionated uncle, four drinks in at a family gathering, playing the "both sides" game on a "there's just one side" kind of issue.
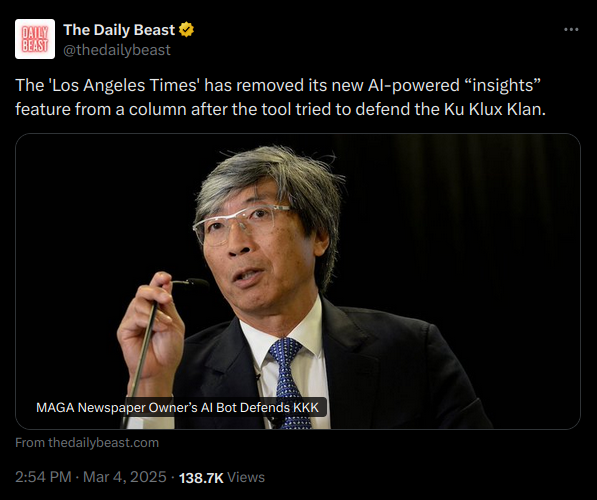
It's far from the worst thing a genAI bot has uttered. Let's not forget the time Google's Bard (predecessor to Gemini) shared extremist views on slavery and genocide. That was two years ago. Google could at least claim that Bard was a pioneer, thereby giving it room to make pioneer mistakes. The LA Times, by comparison, strolled a well-trod path while ignoring the warning signs.
Longtime readers already know what I'm going to say here:
Laugh if you want, but don't forget to check your own work. What are your bots saying in public? And are you okay with that?
Best when used properly
Between the Apple Intelligence summarization goofs, the outspoken LA Times bot, and other genAI mishaps we've witnessed over the past couple of years, I want to be clear:
I don't blame the technology.
The technology is sound. I should know – I've built a career on getting machines to find patterns in data. The problem is when the technology is assigned to duties for which it is ill-qualified. This usually happens when people in leadership roles don't understand AI's capabilities and limits, yet chose to plow ahead on positive vibes. They are unsurprisingly ill-prepared when said vibes fail to make the model behave.
I'm reminded of the much-maligned value-at-risk (VaR) calculation, which has taken a lot of heat in discussions of the 2008 US financial crisis. Yes, VaR has its weaknesses. As does any system. The problem wasn't VaR, though, but that VaR was misused. Misunderstood. Misinterpreted. Banks used it for a quick decision when nuance was the order of the day.
Leadership ignorance is a key source of risk with any tool. Expect AI to provide more such examples over time.
Finding the on-switch
To Apple's credit, they've learned at least one lesson from the headline summarization gaffes: the new prioritization feature is off by default. I'velong said new features are fine; the lack of a clear on-switch is the real problem.
Notice I said on-switch; not off-switch. It's better to tell people about the shiny new toy and let them decide to enable it, rather than enable it by default to artificially fluff your product metrics. By requiring the end-user to switch on the prioritization feature, Apple can fend off most – I emphasize "most," not "all" – criticism of errors. "Hey you chose to activate this! Which means you also know how to deactivate it. So there."
(To qualify my point about "most," not "all": informed consent is key. You have to let people know the full scope of what they're getting into. If you're tricking people into enabling a feature, by vastly overstating its capabilities, that's just as bad as enabling it by default and hiding the off-switch.)
Trusting people
If the machines need extra supervision, does that mean people are more trustworthy? Maybe.
You might see otherwise if you've heard about Citigroup's $18tn (yes, "trillion") near-miss of an internal transfer. (I first found this in the Financial Times. If your FT subscription has lapsed, CNN also provides a rundown.)
The good news is that someone caught the erroneous transfer before it got too far. The bad news is that the almost-transfer had passed through a couple levels of human checks before being caught.
This is when you might call for machines to take over that task, as an automated system would not have made such a mistake. Doubtful. Remember that humans build and program those machines, so it's likely that an automated system would have blown past similar risk controls. Worse still, machine errors occur at machine speeds. And at machine scale. Anyone who's cool mixing that with "minimal human intervention" has never seen the crypto markets.
No, the lesson here is not to automate everything where a human might slip. The lesson is that, if you must assign a task to a human, give them the right tools. And I'm not convinced that Citi got that memo. Per the linked Financial Times article (emphasis added):
Citi's technology team instructed the payments processing employee to manually input the transactions into a rarely used back-up screen. One quirk of the program was that the amount field came pre-populated with 15 zeros, which the person inputting a transaction needed to delete, something that did not happen.
This is not the first time Citi has made news because of a confusing UI. Remember that time in 2020, when they accidentally paid off $900mn in loans? Similar story. Except that no one caught that money before it left the bank.
So before we play "blame the human," I'd like to bring up an old idea from safety expert Todd Conklin. Loosely paraphrased, he says that you can dramatically improve workplace safety if you make it easy to do things the right way, and make it hard to do things the wrong way.
Applied to Citi's UI/UX goofs, we can frame that as: when it comes to manual tasks, a well-designed UI is an amazing form of risk control.
Coming soon …
I've been jotting down notes on agentic AI, but that segment keeps getting bumped because … the AI space won't stop creating other things I need to write about. I'll have more to say on this topic soon. Hopefully. In the meantime, here's a quick preview of what I'll cover:
- Hype
- Hope
- Interfaces
- Intermediaries
It's not quite "Tinker, Tailor, Soldier, Spy" but it'll do.
In other news …
- The Verge's Elizabeth Lopatto gives ChatGPT a philosophy exam. You want to read this one. Seriously. (The Verge)
- In spite of ("because of?") its run-ins with Apple Intelligence, the BBC is creating its own AI department. (The Guardian)
- Would you book a robot massage? (Bloomberg)
- Some movie studios have turned to AI for dubbing. (Les Echos 🇫🇷)
- With enough prompting, you can get a genAI chatbot to simulate having a personality. What happens when we extend that thinking to autonomous vehicle behavior? (And, how soon till manufacturers claim ownership of a certain style?) (WSJ)
- Your Periodic Reminder That GenAI Chatbots Are Not Suitable Replacements For Search, law firm edition. (Ars Technica)
- It turns out that AI models will cheat on occasion. Instead of "cheating," you can also call it "being hell-bent on fulfilling one's objective function." (MIT Technology Review)
- A student built a genAI tool to blow past FAANG tech interviews. Which tells you everything you need to know about FAANG tech interviews. (Gizmodo)
- If someone ever tells you that word-based blocklists are effective screening tools, you can tell them about the time the word "Luigi" tripped up Reddit's content moderation system. (The Verge)
The wrap-up
This was an issue of Complex Machinery.
Reading online? You can subscribe to get this newsletter in your inbox every time it is published.
Who’s behind Complex Machinery? I'm Q McCallum. I think a lot about AI and risk, which I write about here.
Disclaimer: This newsletter does not constitute professional advice.